How Algorithms Improve Patient Adherence
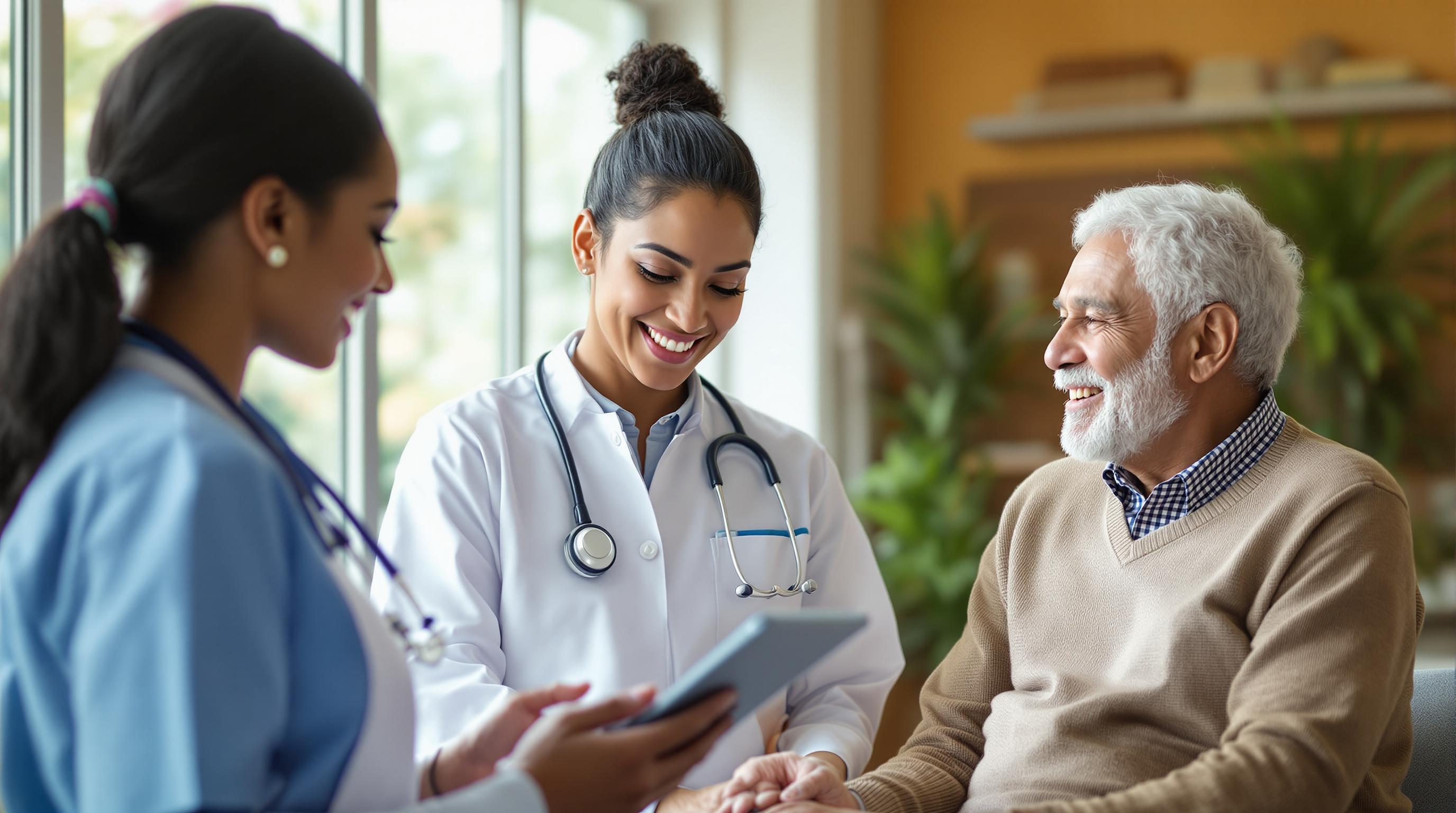

Key Takeaways
How Algorithms Improve Patient Adherence
Nearly half of patients with chronic conditions don’t follow their prescribed treatments, causing $300 billion in healthcare costs annually and 50% of treatment failures. Algorithms are changing this by addressing key barriers like lack of personalization, communication gaps, and psychological challenges. Here's how:
- Personalized Care: Machine learning predicts adherence risks and tailors interventions.
- Mentor Matching: Algorithms pair patients with mentors based on shared traits and needs, improving support.
- Results: Studies show a 25% drop in treatment abandonment and an 18% rise in prescription initiation.
While challenges like system integration and data security remain, these tools are reshaping healthcare by improving adherence and outcomes.
Related video from YouTube
How Mentor Matching Algorithms Work
Mentor matching algorithms help boost patient adherence by connecting patients with mentors who can provide tailored guidance and support. These systems analyze data to create personalized matches that address specific challenges patients face.
Data Used for Matching
To create effective pairings, these algorithms rely on various types of data:
Data Category | Components | Purpose |
---|---|---|
Patient Characteristics | Demographics, Treatment History, Adherence Risks | Identifies patient needs and defines matching criteria |
Mentor-Patient Alignment | Experience, Communication Style, Goals | Ensures mentors can deliver the right type of support |
Techniques Behind the Matching Process
These algorithms use clustering methods to group people based on shared traits. Machine learning models then refine the matching criteria over time, improving the accuracy of pairings by learning from past successes.
"The use of diverse data points and advanced techniques like machine learning can improve the accuracy and effectiveness of mentor matching algorithms" [3].
How Algorithms Tailor Matches
The matching process focuses on personalization through:
- Real-Time Updates: Algorithms adjust matching criteria based on how well current pairings perform, leading to better outcomes for future matches.
- Experience-Based Pairing: Patients are matched with mentors who have dealt with similar challenges, ensuring relevant and effective guidance.
- Customized Support: The system addresses specific barriers, such as lack of emotional support or psychological hurdles, by creating a personalized mentor-patient relationship.
sbb-itb-8f61039
The Effect of Algorithms on Patient Adherence
Research shows that using algorithms for mentor matching can significantly improve how well patients stick to their treatment plans. These tools are proving to be effective in helping achieve better health outcomes.
Study Results
A multi-facility study found that algorithm-driven mentor matching reduced drop-off rates by 25% and boosted prescription initiation by 18% [2]. Healthcare providers using these systems reported noticeable improvements:
Metric | Improvement | Impact |
---|---|---|
Prescription Initiation & Drop-Off Prevention | +18% / -25% | Increased adherence and treatment uptake |
Patient Satisfaction | Noticeable rise | Greater engagement and satisfaction |
These results highlight how algorithmic mentor matching can address adherence issues on a broad scale.
Comparing Methods
When compared to traditional manual strategies, algorithm-based methods clearly stand out. They excel by analyzing large datasets and spotting patterns that might go unnoticed by humans [1]. This data-driven approach offers scalable and tailored solutions to tackle non-adherence by:
- Providing targeted interventions tailored to individual patient needs
- Predicting adherence risks more accurately
- Quickly adjusting to shifts in patient behavior
Example: PatientPartner
PatientPartner serves as a strong example of the benefits of algorithmic matching. The platform uses advanced technology to improve adherence by:
- Making real-time adjustments based on performance data
- Continuously monitoring the dynamics between patients and mentors
Challenges in Implementing Algorithmic Solutions
Algorithmic solutions hold promise for improving patient adherence, but putting them into practice comes with hurdles that need tackling to ensure better treatment outcomes.
System Integration
Bringing algorithmic platforms into existing healthcare systems takes careful planning. It’s not just about the technology; human workflows and processes need attention too. Healthcare providers must ensure these platforms work smoothly with existing Electronic Health Records (EHR) systems.
Integration Component | Challenge | Solution Approach |
---|---|---|
Technical Infrastructure | Compatibility with older systems | Gradual implementation using middleware |
Data Flow | Handling multiple data sources | Creating a unified data framework |
Staff Adoption | Adjusting to new workflows | Focused training programs |
While technical integration is a big piece of the puzzle, regulatory compliance introduces another layer of difficulty.
Regulatory Compliance and Data Security
Strict regulations like HIPAA and GDPR demand healthcare organizations take strong measures to protect patient data. This includes secure storage, encryption, and regular audits.
"Ensuring regulatory compliance involves adhering to standards such as HIPAA and GDPR, which require robust data protection measures. This includes implementing secure data storage solutions, encrypting patient data, and conducting regular audits to ensure compliance" [2].
Scaling Algorithmic Platforms
As patient numbers grow, maintaining quality and performance becomes critical. Scaling these platforms involves addressing:
- Infrastructure capable of handling larger data loads
- Systems to monitor performance continuously
- Algorithms that can adapt without losing effectiveness
Successfully implementing these solutions means balancing technical demands, strict security protocols, and consistent service quality. Tackling these challenges is key to driving wider adoption and improving patient adherence outcomes.
Conclusion: The Future of Algorithms in Patient Adherence
Main Points
Algorithms are reshaping how healthcare tackles patient adherence by offering precise, tailored interventions. Research shows that predictive algorithms can pinpoint patients at high risk for non-adherence, allowing healthcare providers to offer targeted support [1]. Tools like PatientPartner showcase how algorithm-based solutions can lead to better patient outcomes.
Impact Area | Current Achievement | Future Potential |
---|---|---|
Predictive Accuracy | 50% evidence availability score | Real-time, more accurate predictions |
Patient Engagement | 25% reduction in drop-offs | AI-driven, personalized assistance |
Treatment Efficacy | 18% increase in prescription rates | Integrated clinical decision-making support |
With these existing milestones, upcoming advancements are set to push patient adherence solutions even further.
Future Innovations
Deep learning models are paving the way for real-time tracking and analysis of adherence [1]. These technologies offer the potential for more advanced intervention strategies, as highlighted by experts:
"Integrating predictive analytics with real-time patient data can provide more accurate and timely predictions of adherence risks, enabling healthcare professionals to intervene early and improve patient outcomes" [1].
AI-powered platforms that combine predictive analytics with interactive tools are becoming essential in delivering personalized care. As machine learning evolves, we’ll likely see these algorithms applied across a broader range of conditions, improving their ability to predict and address non-adherence [4].
While challenges like scaling and ensuring data security remain, these advancements position algorithms as a cornerstone of future healthcare. By offering more responsive and tailored support, they hold the promise of significantly improving patient outcomes.
Author
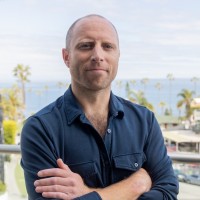
Co-Founder and CEO of PatientPartner, a health technology platform that is creating a new type of patient experience for those going through surgery