Using Sentiment Data to Predict Patient Drop-Off Risks
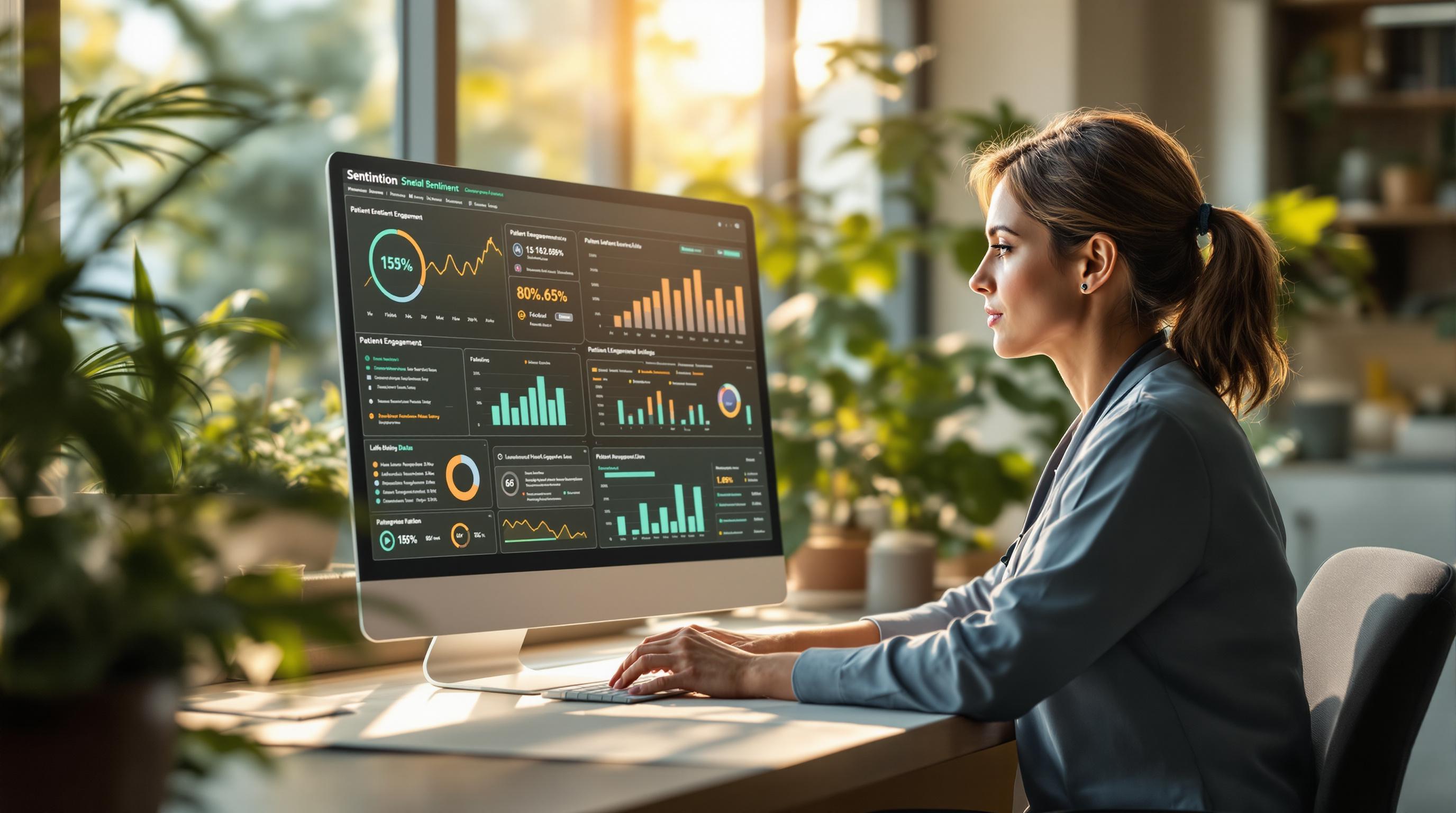

Key Takeaways
Using Sentiment Data to Predict Patient Drop-Off Risks
Patient drop-offs are a big problem in healthcare, leading to worse health outcomes, lost revenue, and inefficient operations. But sentiment data - analyzing patient emotions and feedback - can help predict and prevent these risks. Here's how:
- What causes drop-offs? Patients stop following treatments due to dissatisfaction, frustration, or lack of engagement.
- Why current methods fail: Traditional solutions miss early warning signs and lack personalized, real-time interventions.
- How sentiment data helps: By analyzing surveys, reviews, and communication patterns, healthcare providers can predict disengagement and act early.
Key benefits of using sentiment data and predictive analytics:
- 33% fewer drop-offs: Real-time tools like PatientPartner track patient emotions and provide mentorship.
- Better patient experience: Tailored care improves satisfaction and adherence.
- Operational efficiency: Early risk detection reduces wasted resources and staff workload.
Sentiment analysis isn't just about data; it's about understanding and responding to patients' needs in real time for better outcomes.
Sentiment analysis in health care – an overview
Using Sentiment Data in Predictive Analytics
Healthcare organizations are using sentiment data to better predict and prevent patient drop-offs. By identifying emotional triggers, this data helps providers step in early to keep patients engaged.
What Is Sentiment Data?
Sentiment data reflects patients' feelings and attitudes about their healthcare experiences. A major source is the Hospital Consumer Assessment of Healthcare Providers and Systems (HCAHPS) survey. Other sources include patient feedback forms, emails, phone calls, and online reviews [1].
Data Source Type | Examples | Key Insights Captured |
---|---|---|
Structured | HCAHPS surveys, satisfaction ratings | Satisfaction levels |
Semi-structured | Electronic health records (EHRs) | Patterns in treatment adherence |
Unstructured | Patient communications, online reviews | Emotional feedback |
How Sentiment Analytics Identifies Drop-Off Risks
Through language analysis, sentiment analytics tools can detect early signs of disengagement [2].
"Sentiment analysis allows healthcare providers to predict disengagement by understanding patient perceptions of processes, treatments, and care teams."
Machine learning models analyze patterns to flag potential drop-offs. Here are some of the key areas they monitor:
- Interaction patterns: Shifts in tone, communication frequency, or follow-up behavior
- Treatment satisfaction: Concerns about procedures or medications
- Emotional signals: Words or phrases that indicate frustration or dissatisfaction
Real-time patient support platforms further enhance this process. For instance, mentorship programs that gather immediate feedback can quickly identify and address patient concerns [3].
Steps to Implement Predictive Analytics
Collecting and Integrating Data
The first step in predictive analytics is bringing together data from various sources like EHR systems, surveys, social media, and real-time support tools. Before this data can be useful, it needs to go through preprocessing steps such as cleaning, preparation, and normalization. This ensures the data is accurate and ready for analysis. At the same time, maintaining HIPAA compliance and high data quality is crucial throughout this process.
Once the data is integrated and prepared, it sets the stage for building predictive models to uncover insights and guide actions.
Developing Predictive Models
Predictive models allow healthcare providers to spot and address potential patient drop-off risks before they occur. To create these models, you’ll need to identify key risk factors, select the right algorithms, and test the models against historical data to ensure accuracy. Algorithms like logistic regression work well for simple predictions, while machine learning techniques are better suited for more complex analyses.
When combined with real-time tools, these models can provide actionable insights and enable immediate responses to patient needs.
Using Real-Time Patient Support Platforms
Real-time platforms take predictive analytics to the next level by using sentiment data to trigger timely interventions. For example, PatientPartner’s platform integrates sentiment tracking with real-time mentorship, helping healthcare providers detect risks early and take swift action to improve patient retention.
To maintain trust, healthcare organizations should routinely review their systems for ethical practices and ensure patients are informed about how their data is being used. Transparency and accountability are key when employing these advanced tools.
sbb-itb-8f61039
Advantages of Sentiment Data and Predictive Analytics
Using predictive analytics alongside sentiment data delivers clear benefits in areas like retention, patient experience, and operational efficiency.
Better Patient Retention
PatientPartner's mentorship program, which incorporates sentiment tracking, has led to a 33% reduction in patient drop-off rates. This approach combines predictive analytics with personalized support, connecting patients to mentors who have firsthand experience with similar journeys. The results speak for themselves: an 85% success rate in helping patients move forward in their care process [3].
Improved Patient Experience
Sentiment-driven care tailors healthcare to individual needs while improving responsiveness. Companies like Eli Lilly and Pfizer use this approach to address patient concerns proactively, which boosts satisfaction and adherence [3].
"Sentiment analysis allows healthcare organizations to analyze patient reviews, comments, and surveys to gain a deeper understanding of patient sentiments." - Voxco [6]
Increased Efficiency
Predictive analytics streamlines resource allocation by identifying high-risk patients early, allowing for timely interventions. This not only reduces the need for manual monitoring but also enhances staff efficiency. Machine learning tools that analyze patient feedback have been especially useful in evaluating hospital performance [4]. Real-time monitoring and insights further improve engagement while lightening the workload for staff.
These operational improvements enhance current processes and set the stage for advancements in patient care.
Future Developments and Innovations
The field of sentiment data and predictive analytics in healthcare is changing fast, thanks to new technology and a growing emphasis on patient-focused care.
AI and Machine Learning Transforming Healthcare
Deep learning and natural language processing (NLP) are reshaping how healthcare providers understand patient behavior. These tools allow for detailed analysis of diverse data sources, like electronic health records, social media, and patient surveys.
"Sentiment analysis offers real-time insights into patients' emotions, enabling personalized care." - Binariks [5]
Today, advanced AI systems can analyze data from wearables, social media, patient feedback, and clinical records to spot adherence issues and flag potential drop-off risks earlier than ever before. By combining real-time health tracking with sentiment analysis, these systems provide a full picture of patient engagement [1].
This shift in technology is paving the way for more responsive platforms that address patient needs and risks as they arise [2].
Evolving Patient-Centered Platforms
With AI insights at the core, healthcare platforms are becoming better at offering tailored support. These platforms now merge sentiment data with clinical information to deliver real-time, personalized care for patients at higher risk.
Some key advancements include:
- Transparent AI predictions that providers can easily understand and trust
- Combining clinical data with sentiment analysis for more accurate risk detection
- Enhanced privacy measures to ensure secure data handling
Healthcare providers and pharmaceutical companies are working together to create better patient engagement strategies. By standardizing data collection and analysis methods, these collaborations aim to turn technological progress into tangible improvements in patient care - all while keeping privacy a top priority [4].
As sentiment analysis and predictive analytics continue to develop, the focus remains on clear, patient-first solutions that build trust and reduce patient drop-offs effectively.
Conclusion: The Role of Sentiment Data in Healthcare
Sentiment data and predictive analytics are proving to be essential in tackling a major issue in healthcare: patients discontinuing treatment. These tools help healthcare providers spot risks early and take action to keep patients on track. This shift toward focusing on patient needs highlights how sentiment data can play a key role in predicting and addressing drop-off risks.
By analyzing patient feedback and emotions, sentiment-based models can pinpoint potential issues before they escalate. This allows healthcare providers to step in with personalized solutions, reducing drop-offs and improving overall care. The results? Better outcomes, earlier risk detection, and more tailored interventions.
As healthcare evolves, understanding and responding to patient sentiments in real-time will become even more important. Combining AI with sentiment analysis enables systems that react faster and meet patient needs more effectively.
Using sentiment analysis isn’t just about gathering data - it’s about creating a healthcare system that listens and responds. By integrating these tools into their processes, organizations can better align care with patient needs, reduce drop-offs, and provide more effective, compassionate care through timely, data-informed actions.
Author
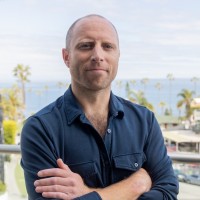
Co-Founder and CEO of PatientPartner, a health technology platform that is creating a new type of patient experience for those going through surgery