How Algorithms Personalize Mentor-Patient Support
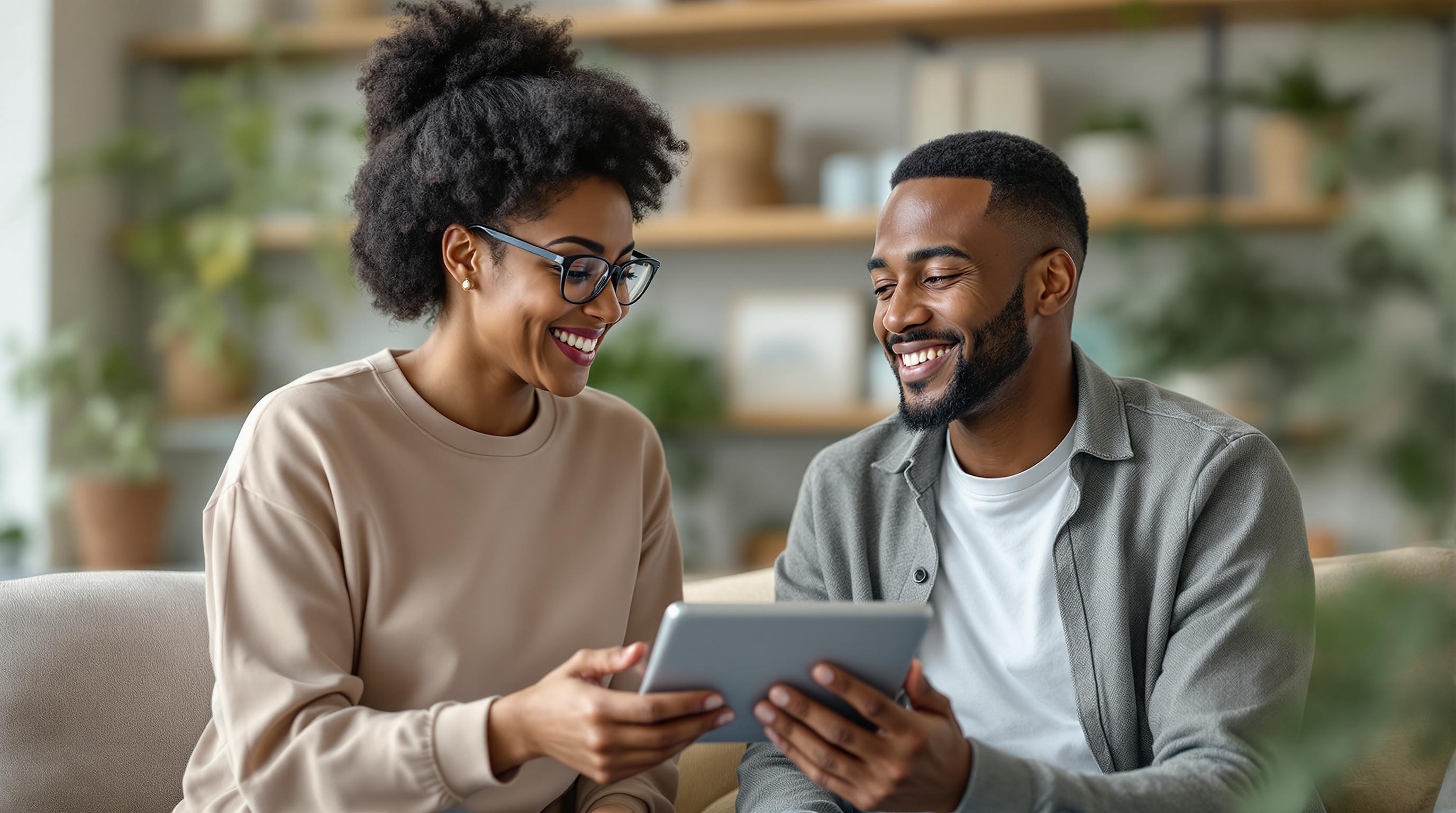

Key Takeaways
How Algorithms Personalize Mentor-Patient Support
This personalized approach improves treatment adherence, boosts prescription uptake, and enhances patient support.
Key Highlights:
- 25% drop in adherence issues and 18% rise in prescription rates through tailored mentor-patient programs.
- AI systems analyze medical history, demographics, and preferences to match patients with compatible mentors.
- 85% success rate in helping patients progress in care through platforms like PatientPartner.
- Data privacy is ensured with encryption, access controls, and de-identification.
Why It Matters:
Mentor-patient matching fills gaps in standard care by offering emotional and practical support, improving outcomes, and ensuring better engagement. AI-driven tools refine these matches over time, ensuring strong, meaningful connections.
Related video from YouTube
Patient Data Used in Mentor Matching
Algorithms use key data points to create personalized matches tailored to each patient’s unique needs.
Core Patient Matching Data Points
Modern mentor matching systems rely on detailed patient profiles to build meaningful connections. These algorithms assess various factors to pair patients with mentors who can provide the most support.
Here are the main data points used:
Data Category | Specific Elements | Purpose |
---|---|---|
Medical Profile | Medical history, current treatments | Ensures mentors have relevant experience |
Demographics | Age, gender, location | Builds relatable connections |
Treatment Journey | Treatment stage, upcoming procedures | Matches patients with mentors who've had similar experiences |
Personal Preferences | Communication style, availability | Enhances engagement and interaction |
Integration with electronic health records (EHR) ensures profiles stay accurate and up-to-date, allowing for real-time match adjustments as needed.
Data Privacy and Security Standards
Protecting patient data is essential, and strict measures are in place to ensure compliance with regulations like HIPAA and GDPR.
Key security measures include:
- Encrypted data storage and secure APIs: Safeguards information during storage and transfer.
- Access controls with audit trails: Tracks and limits who can access sensitive data.
- De-identification of data: Strips personal identifiers before processing to maintain privacy.
PatientPartner’s platform stands out in this area, applying advanced security protocols that have contributed to an 85% success rate in helping patients progress through their care journey [2].
Building Effective Matching Algorithms
Today's mentor-patient matching systems combine advanced data analysis with human oversight to create connections that matter. These algorithms use multiple layers of processing to support patients throughout their healthcare journey.
Setting Match Criteria
The data points mentioned earlier form the backbone of these criteria, ensuring matches are both medically relevant and personally meaningful. The algorithms prioritize specific characteristics based on medical and personal factors.
Priority Level | Matching Criteria | Impact on Outcomes |
---|---|---|
Primary | Medical condition, treatment experience, and stage/timeline | Direct influence on treatment adherence |
Secondary | Communication preferences & availability | Enhances engagement quality |
Tertiary | Demographics & location | Boosts relatability |
Once the criteria are set, machine learning steps in to fine-tune the process by analyzing patterns and results.
Machine Learning in Match Optimization
Machine learning plays a key role by improving matching accuracy through pattern recognition and outcome analysis. For example, PatientPartner's platform has achieved an 85% success rate in helping patients move forward in their care journey [2]. The system learns from factors like patient engagement, adherence rates, interaction quality, and health outcomes to refine its matches over time.
Human Review in Algorithm Decisions
Although algorithms handle the initial match, healthcare professionals step in to review and adjust. They verify medical accuracy, evaluate emotional compatibility, and ensure mentors have the right experience. This is especially important for rare conditions or unique patient needs, where manual adjustments may be necessary.
sbb-itb-8f61039
Patient Engagement Through Matched Mentors
AI-powered platforms are transforming how mentors and patients connect, helping improve treatment adherence and outcomes through personalized support.
Building Patient-Mentor Trust
After mentors and patients are paired, trust becomes the foundation of their relationship. By using insights from patient profiles, mentors can tailor their communication, which has led to an 85% success rate in advancing patient care [2].
Trust-Building Factor | Impact on Engagement |
---|---|
Shared Medical Experience and Timeline Alignment | Boosts credibility and ensures timely, relevant guidance |
Communication Style Match | Creates a sense of ease and openness |
Cultural/Demographic Fit | Deepens the personal bond between mentor and patient |
Real-Time Support Adjustments
AI tools continuously monitor how patients engage and progress, helping mentors fine-tune their approach. This allows mentors to respond quickly to changes, ensuring the support remains effective. PatientPartner is a standout example, using real-time insights to strengthen mentor-patient connections and deliver better outcomes.
PatientPartner's Mentor Matching System
PatientPartner’s platform showcases how AI can revolutionize patient support. By combining smart matching algorithms with ongoing progress tracking, it ensures mentors and patients form strong, productive relationships. This system has led to a 25% reduction in adherence drop-off rates and an 18% increase in prescription rates, thanks to tailored mentor strategies.
The platform is designed with strict HIPAA and SOC 2 compliance in mind. Mentors receive real-time updates about patient progress and engagement, enabling them to make informed decisions about how to adjust their support.
"PatientPartner's platform uses AI to track patient progress and adjust mentor support accordingly, resulting in a 25% reduction in patient adherence drop-off rates and an average 18% lift in prescription rates" [4].
Measuring Algorithm Performance
Evaluating and improving mentor-patient matching requires a focused approach to data analysis and ongoing updates. Healthcare organizations rely on specific metrics to assess how effectively their algorithms pair patients with the right mentors.
Success Metrics
The success of mentor-patient matching algorithms is tracked using several performance indicators that directly influence patient outcomes.
Metric Category | Performance Indicators & Impact |
---|---|
Treatment Adherence | Prescription fill rates and compliance, resulting in a 25% drop-off reduction |
Patient Engagement | Metrics like session participation and communication frequency |
Clinical Outcomes | Treatment completion rates and measurable health improvements |
Program Satisfaction | Feedback from patient and mentor satisfaction surveys |
These metrics are typically reviewed on a quarterly basis to ensure matching algorithms perform effectively.
Using Feedback to Improve Matches
Improving algorithms relies heavily on structured feedback from both patients and mentors. Organizations collect this feedback through surveys, regular check-ins, and milestone reviews. The insights gained help refine the matching process, making it more accurate and effective over time.
Patient feedback highlights the quality and success of matches, while mentors provide input that helps fine-tune criteria and identify areas for improvement. For example, PatientPartner uses insights from its network of over 500 Patient Ambassadors to enhance its proprietary matching algorithm [4].
Consistent updates informed by feedback have shown measurable improvements in patient outcomes. Organizations using these methods report stronger connections between mentors and patients, as well as higher treatment adherence rates. Striking the right balance between automated processes and human oversight remains crucial for achieving the best results.
Conclusion: Next Steps in Mentor-Patient Support
Algorithm-driven mentor matching has transformed patient support by boosting adherence and delivering better outcomes. With the predictive analytics market projected to reach $126.15 billion by 2032 [1], there's clear momentum toward using AI to enhance healthcare personalization.
For healthcare organizations adopting these systems, focusing on specific priorities will help ensure they remain effective and scalable.
Priority Area | Key Actions | Expected Benefits |
---|---|---|
Technology Infrastructure | Integrate secure platforms with strong data protection | Improved scalability and compliance |
Algorithm Development | Implement and fine-tune advanced machine learning models | More precise matches and better outcomes |
Mentor Network Growth | Expand mentor networks strategically | Broader patient access and support |
The financial benefits of improved adherence are also striking. Just a 10% rise in adherence could generate an additional $41 billion in revenue for U.S. pharmaceutical companies while cutting into the $100 billion annual cost of non-adherence [3].
Aravind Chandrasekaran highlights the importance of patient involvement:
"Patients and former patients can and should be co-creators of value in the transition of care process" [3].
To move forward, healthcare organizations must focus on integrating secure, scalable technology, refining algorithms through feedback, and using clear metrics to track results. As these systems evolve, the ability to personalize and scale will remain essential, ensuring patients receive high-quality, targeted mentor support that drives meaningful engagement and better outcomes.
FAQs
What is the most important factor when matching a mentor?
When it comes to mentor-patient matching, relevant treatment experience stands out as the most important factor. Mentors with firsthand knowledge can offer practical advice and build trust, making the support relationship more effective.
Here are three factors that influence successful matches:
Factor | Importance | Impact |
---|---|---|
Treatment Experience | Primary | Provides direct insight into specific procedures and challenges. |
Disease State Knowledge | High | Helps mentors connect over shared symptoms and management approaches. |
Communication Style | Essential | Builds trust and ensures meaningful engagement. |
Technology, particularly algorithms, helps identify mentors with the right experience, ensuring matches are accurate and beneficial. Research indicates that shared treatment experiences significantly boost engagement and improve outcomes [1].
PatientPartner demonstrates this concept with its advanced matching system. Their platform pairs patients with mentors who have faced similar treatments, successfully connecting thousands of people across a wide range of medical procedures [2].
Author
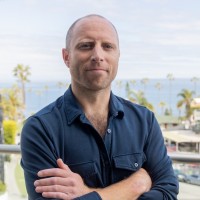
Co-Founder and CEO of PatientPartner, a health technology platform that is creating a new type of patient experience for those going through surgery